教育部人文社科重点研究基地中央财经大学中国精算研究院学术活动
精算论坛第262期讲座
(2025年5月14日)
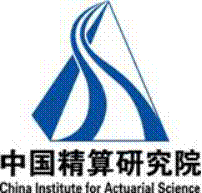
讲座主题一:Pricing financial and insurance derivatives within parameter uncertainty
摘要:In this talk, we consider two different papers that explore the applications of robust pricing within the Uncertain Volatility Model (UVM) in the fields of finance and insurance.
In the first paper [1] we consider the application of Machine Learning techniques for pricing high- dimensional options within the framework of the UVM. The UVM is a robust framework that accounts for the inherent unpredictability of market volatility by setting upper and lower bounds on volatility and the correlation among underlying assets. By leveraging historical data and extreme values of estimated volatilities and correlations, the model establishes a confidence interval for future volatility and correlations, thus providing a more realistic approach to option pricing. By integrating advanced Machine Learning algorithms, we aim to enhance the accuracy and efficiency of option pricing under the UVM, especially when the option price depends on a large number of variables, such as in basket or path-dependent options. Our approach evolves backward in time, dynamically selecting at each time step the most expensive volatility and correlation for each market state. Specifically, it identifies the particular values of volatility and correlation that maximize the expected option value at the next time step, and therefore, an optimization problem must be solved. This is achieved through the use of Gaussian Process regression, the computation of expectations via a single step of a multidimensional tree and the Sequential Quadratic Programming optimization algorithm. The numerical results demonstrate that the proposed approach can significantly improve the precision of option pricing and risk management strategies compared with methods already in the literature, particularly in high-dimensional contexts. In the second paper[2], we propose a novel methodology for pricing equity-indexed annuities featuring cliquet-style payoff structures and early surrender risk, using advanced financial modeling techniques. Specifically, the market is modeled by an equity index that follows an uncertain volatility framework, while the dynamics of the interest rate are captured by the Hull-White model. Due to the inherent complexity of the market dynamics under consideration, we develop a numerical algorithm that employs a tree-based framework to discretize both the interest rate and the underlying equity index, enhanced with local volatility optimization. The proposed algorithm is compared with a machine learning-based algorithm. Extensive numerical experiments demonstrate its high effectiveness. Furthermore, the numerical framework is employed to analyze key features of the insurance contract, including the delineate on of the optimal exercise region when early surrender risk is incorporated.
报告人:Antonino Zanette
Antonino Zanette is a Full Professor in Mathematics for Economics at UdineUniversity (Italy), author of numerous publications in leading journals of mathematical finance and scientific leader from 1998 to now of the software project Premia (a computational platform designed to set up a technology watch for numerical problems related to the evaluation of financial derivative) of the INRIA Paris MathRisk project (www.premia.fr\rm). Hismainly research activity is in Computational Finance. The main topics are: tree methods for exotic American options, insurance derivatives in Black-Scholes, stochastic volatility and jumps models. Variables Annuities, finite difference methods for pricing American options on two stocks, American lookback options, Swing options. Monte Carlo methods for pricing and hedging American option in high dimension machine learning for finance and insurance.
讲座主题二:On improving interpretability of artificial neural networks in applications to computational finance
摘要:We propose probabilistic analogs of the universal approximation theorems and link continuous random variables of a certain type and monotonic feedforwardartificial neural networks with one-dimensional input, output and one hidden layer. In particular,we show that any continuous infinitely divisible random variable can be successfully approximated with a mix of logistic distributions. Based on the probabilistic analogs of the universal approximation theorems,we develop a new approach for designing Monte Carlo methods combined with artificial neural networks for pricing options in Levy models.
We demonstrate how to improve the interpretability of neural networks using standard problems of computational finance as examples.
报告人:Oleg Kudriavtsev
Dr. Kudriavtsev is the Head of the Department of Computer Science and Customs Technologies at the Rostov Branch of Russian Customs Academy and Professor at the Institute of Mathematics, Mechanics and Computer Science of Southern Federal University and Research Director at InWise Systems, LLC. He is an expert in Computational Finance and Applied Mathematics, a member of the international research group MathRisk. Oleg Kudriavtsev holds a Degree of Doctor of Science in Physics and Mathematics (Russian analog of Habilitation Degree) from Central Economics and Mathematics Institute of Russian Academy of Science, (Moscow, Russia). The field of his research interests includes the development of fast and efficient computational algorithms for pricing path-dependent options and risk estimation in models admitting jumps (numerical Wiener-Hopf factorization, Monte Carlo methods, finite difference schemes, integral transform methods).
讲座时间:2025年5月14日(周三)
上午14:00-16:00
报告地点:沙河校区学院楼13号楼215
邀 请 人:韦晓